First off, why mess with such a retro format as Arc/Info Export (.e00)?- any code written for this ASCII file type in the last few years has been on how to go from e00 to pretty much anything (especially to the non-topological data format, the shapefile).
Put simply, topological information makes a lot of things possible for the intrepid ActionScripter.
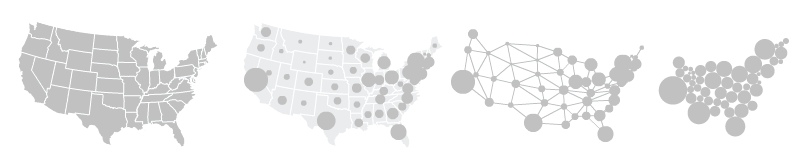
E00 files non-redundantly store all nodes, lines, and polygons that make up a geographic data layer. This geodata format is one of three currently distributed by the Census Bureau for boundary files (the others are the shapefile and the Ungenerate ASCII format). The GIS formats used in most web mapping applications (I’m thinking of shapefiles, GeoJSON, and KML) are non-topological, meaning features are stored independently, and topological information on shared borders and the like is quite difficult to extract. Like seriously hard. Something you don’t want to be doing in the browser. Matthew Bloch, of the NY Times, did his cartography master’s thesis (at Wisconsin, natch) on MapShaper, much of which involved a C++ server-side solution for building topology from a polygonal shapefile. Generalization requires non-redundant polylines so as not to create gaps between features when smoothing. Other visualization techniques, including cartogram construction and graph decomposition, also require knowing the shared borders of geographic features.
Ideally, such topological information could be created/extracted for any geography, regardless of the datasource. In reality, topology building is intensive and best suited to server-side processing. Using E00 files and my E00Parser lets you experiment with the visualization and cartographic techniques only possible when such topological information is known, without the expensive processing necessary to build it.
The code
I’ve gotten a ton of use out of Edwin van Rijkom’s SHP library. My noncontiguous cartogram, isolining, and political choropleth experiments relied on the code to load coordinate data in shapefile form at run-time, as did the early experiments that led to indiemapper. I’m hoping I’ll get just as much use out of this parser, for when adjacency information is critical to the visualization technique.
There are two main classes, E00Parser and E00Tools. E00Parser is based on the Perl extension Geo::E00 by Alessandro Zummo and Bert Tijhuis, with much aid from the (world famous) Arc/Info Export Format Analysis. There’s no way I would have attempted to write the AS3 E00 parser without Zummo and Tijhuis’ code, as theirs appears to be the only stand-alone open source code available for reading the format. Their Perl regular expressions were copied with few modifications, though I did fix an issue in some that was keeping their code from accurately reading certain sections of double-precision coverages. I wrote E00Tools to collect a handful of methods for working with the resultant data.
I setup a Google Code project for this work, as topology will likely form the basis for a decent amount of my cartographic experimentation in the near future.
- to browse the code, just go here
- the latest zip distribution is available here
- three examples are included in com.indiemaps.mapping.data.parsers.e00.examples
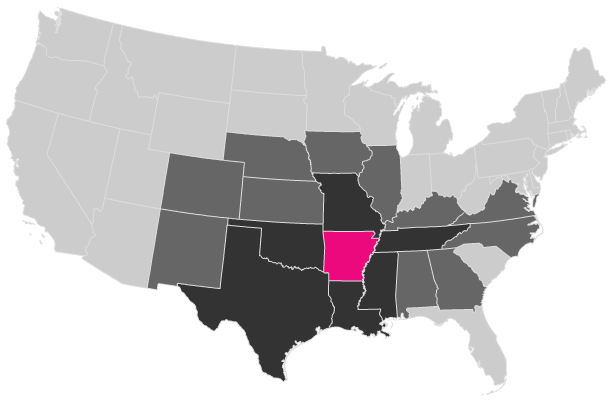
Oh, BTW:
ESRI considers the export/import file format to be proprietary. As a consequence, the identified format can only constitute a “best guess” and must always be considered as tentative and subject to revision, as more is learned.
(from the Arc/Info Export Format Analysis)
How to use
After loading your ASCII E00 file into a string, use something like the following to parse it.
The returned data object includes all information contained in the file, and can have as many as nine sections. Of most use are the arc (non-redundant list of polylines), pal (list of all polygons and their associated lines), and ifo (attributes and labels) sections. The exact structure of the returned object is described on the wiki here.
There are three sweet examples to be found in com.indiemaps.mapping.data.parsers.e00.examples.
Tools
E00Tools contains some methods for working with the resultant data of E00Parser.parse(). Included are methods for:
- Drawing all features
- Drawing individual features
- Getting a list of polygon IDs for all features
- Getting the centroid of a feature
- Getting the shared border length of all features and their neighbors
Key above is the idea of the feature. Michigan is a feature. Features are not directly encoded in E00 files like they are in other formats. In a polygonal shapefile, for example, each feature is encoded as a multipolygon, constituted of one or more rings of points. In E00 files, only polygons are directly encoded; feature information (which polygons make up which features) can be ascertained from the INFO (ifo) section.
Experimentation
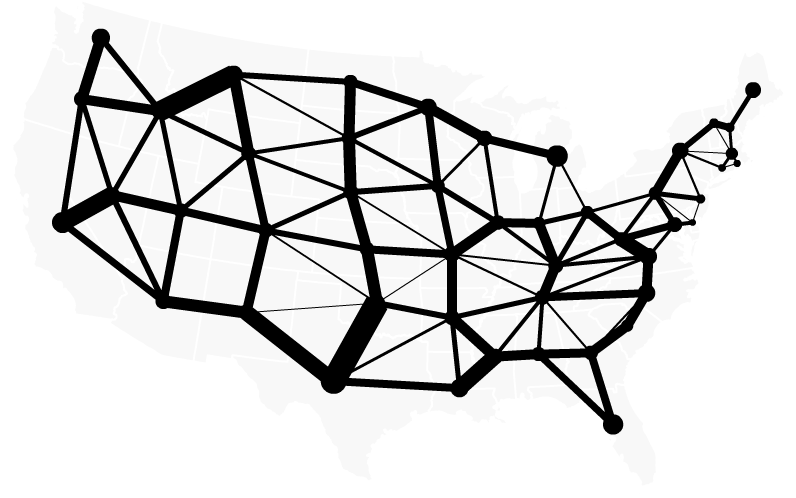
I created these AS3 classes for myself, because I wanted to experiment with topological geodata in visualization and cartographic applications. This typically boils down to knowing which features are neighbors and how much of a border they share. The E00Tools methods getAllFeatureNeighbors and getAllFeatureSharedBorderLengths gives you easy access to this information.
Daniel Dorling popularized the circular cartogram form among academic cartographers, outlining the symbology most notably in his 1991 PhD thesis and 1996’s Area Cartograms: Their Use and Creation (available here in PDF form along with many other gems of quantitative geography). Dr. Dorling made Pascal and C code available. I ported it to Python, and began experimenting, mostly in vain, on a method that worked with a shapefile as input, but without the expense of building topology. It produced at best a pale imitation. Dorling describes the gravity model used to produce the cartograms in his dissertation:
The algorithm which was developed to create the area cartograms worked by repeatedly applying a series of forces to the circles representing the places. Circles attract those they are topologically adjacent to; the strength of this attraction being greater the larger the distance is between them and the longer their common boundary.
The algorithm thus requires the shared border lengths of all features and their neighbors. Producing this info is easy with E00Tools, but it seems kind of backward to parse my geodata in ActionScript only to produce the rendering in Python. I’m working on porting Dorling’s algorithm to AS3 so I can go directly from geodata to cartogram without switching platforms.
Lee Byron mentions another technique, used to generate the Olympic Medal Count cartograms he helped produce for the Times. Byron didn’t release any code, but notes that a soft body force directed layout algorithm written in ActionScript was used. I haven’t been able to reproduce his method, but I’ve included an example that drops the topological information gathered from an E00 file into a Flare visualization using a force directed layout. The example is minimal, but shows how the E00 classes can be integrated with the Flare visualization API, and may point the way to a slightly different method for producing circular cartograms client-side.